In the world of data, consistency is king. It's the backbone of reliable analysis and decision-making.
But what happens when data becomes inconsistent?
Inconsistent data can disrupt your operations, leading to faulty insights, poor customer experiences, and even regulatory non-compliance.
In this article, we'll explore inconsistent data, explaining what it is, why it happens, and, most importantly, how to resolve it.
What is Inconsistent Data?
As the name suggests, inconsistent data refers to data that lacks uniformity or agreement. It occurs when the same piece of information shows up differently in different places.
For instance, a customer's address might be recorded as "123 Main St" in one system and "123 Main Street" in another. This example is simple, but inconsistencies can be more complex and challenging.
Why Does Data Become Inconsistent?
Data inconsistency can creep in for a variety of reasons. When entering data manually, people often have to make corrections. .
Different systems may have different formats or standards for data entry, and integration issues can arise when these systems don't communicate well.
Here are a few common causes of data inconsistency:
- Human error during data entry
- System errors or glitches
- Poorly integrated systems
- Lack of data standards or guidelines
- Changes in data over time
The Impact of Inconsistent Data on Businesses
Inconsistent data can have severe consequences for businesses. However, consistent data can lead to better analysis and better decision-making.
For example, if your sales data is inconsistent, you might underestimate your revenue and make faulty strategic decisions. Inconsistent data can also erode customer trust, especially if it leads to errors in billing or service delivery.
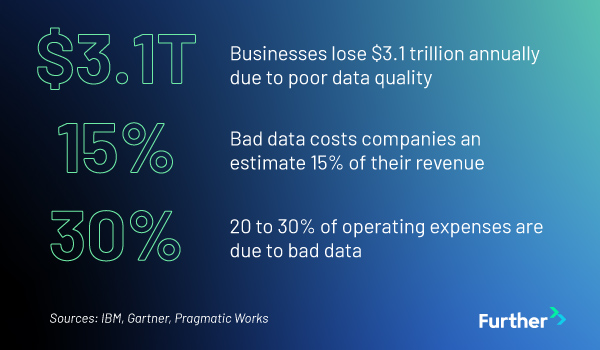
Identifying Inconsistent Data
Spotting inconsistent data can be a challenge. It's like finding a needle in a haystack, especially when dealing with large datasets.
However, some strategies can help. For instance, data profiling can give you a good sense of your data's quality and highlight potential inconsistencies.
Tools and Techniques for Detecting Inconsistencies
Many tools and techniques are available to help detect data inconsistencies. Automated data quality tools can scan your data and flag potential issues.
These tools often include features like:
- Pattern recognition to spot anomalies
- Rule-based checks for data validity
- Cross-field validation to ensure data consistency across related fields
- Data profiling to understand the nature of your data and its inconsistencies
Remember, these tools are just aids. They can't replace a thorough understanding of your data and its context.
Strategies for Resolving Data Inconsistencies
Once you've identified inconsistent data, the next step is to resolve it. This process can be complex and time-consuming, but it's crucial for maintaining data quality.
You can use several strategies, including data cleaning, standardization, and validation. Let's dive into each of these in more detail.
Data Cleaning and Standardization
Data cleaning involves correcting or removing the inconsistent parts of your data. It could mean fixing typos, filling in missing values, or removing duplicates.
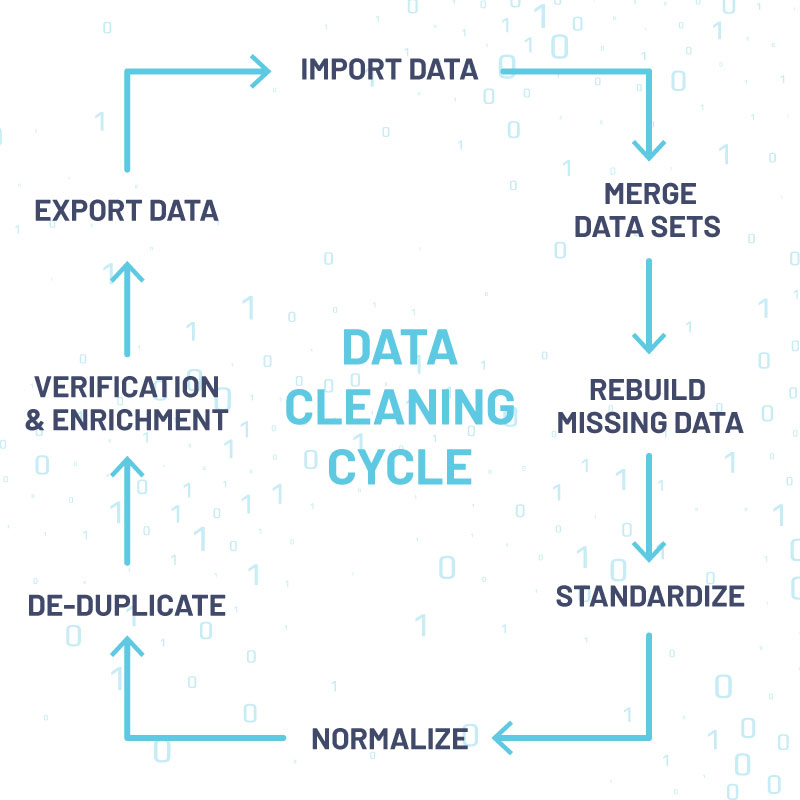
Standardization, on the other hand, is about making your data consistent. Standardization could involve:
- Converting data to a common format
- Aligning data with predefined standards
- Normalizing data to reduce redundancy
Both cleaning and standardization are essential for resolving data inconsistencies.
Implementing Data Validation Processes
Data validation is another crucial strategy for dealing with inconsistent data. It involves checking your data against predefined rules or standards to ensure it's correct and consistent.
Here are some validation techniques you might consider:
- Range checks to ensure data falls within a specific range
- Consistency checks to ensure data is logical and coherent
- Completeness checks to ensure all necessary data is present
Remember, validation should be an ongoing process, not a one-time event. Regular validation can help you catch inconsistencies early and prevent them from causing problems.
Preventing Future Data Inconsistencies
Resolving inconsistent data is just half the battle. The other half is preventing future inconsistencies from cropping up. Prevention requires a proactive approach to data management.
One effective strategy is to implement clear data entry guidelines. Guidelines can help prevent human errors, a common cause of data inconsistencies. It's also important to regularly audit your data to catch inconsistencies before they become significant issues.
Establishing Data Governance and Quality Standards
Data governance is key to preventing data inconsistencies. It involves establishing clear policies and procedures for how data is collected, stored, and used.
Here are some steps you can take to establish strong data governance:
- Define clear roles and responsibilities for data management
- Implement data quality standards and ensure they are adhered to
- Regularly review and update your data governance policies
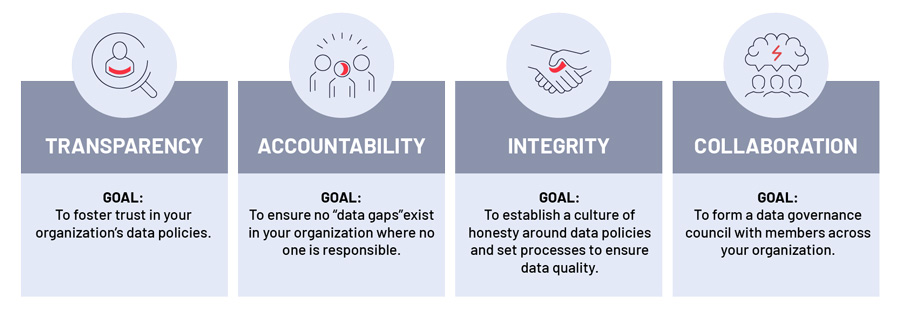
By establishing strong data governance, you can ensure your data is consistently high-quality and reliable. This is not just a theoretical concept, but a practical necessity for businesses today. Data governance can lead to better decision-making and improved business outcomes, making it a crucial step in your data management journey.
Conclusion: The Continuous Journey of Data Quality
Inconsistent data can be a significant roadblock for businesses. But with the right strategies and tools, it can be tackled effectively. Remember, maintaining data quality is not a one-time task, but a continuous journey.
Implementing the strategies discussed in this article ensures your data is accurate, reliable, and consistent. These strategies will improve your decision-making process and boost your business's overall performance. As data professionals, your role is crucial in this process. You are the ones who understand the data and its context, and you are the ones who can use the tools and techniques discussed here to identify and resolve data inconsistencies. Further can jumpstart your journey towards better data quality; contact us today, it's a journey worth taking.